Business Challenge
Asset failure causes widespread financial and operational problems for infrastructure asset-rich industries, for example water, energy and transport. All over the world these industries are under intense pressure from governments, regulators and the public to do more with less whilst maintaining if not improving their safety, reliability and environmental performance. The challenge for asset managers is to find the asset management policy that minimises the total cost in the trade-off between the risk cost of asset failure and the direct cost of asset maintenance and replacement. This requires:
- mitigating the risk of asset failure, consequential damage, for example flooding, pollution and service interruption, and minimising fines from regulators
- reducing the direct cost of asset maintenance and replacement.
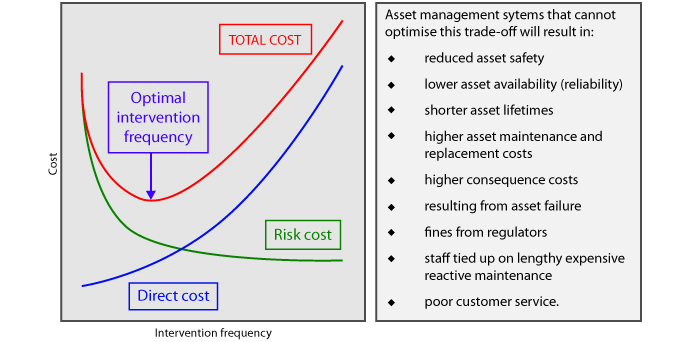
The Solution - PAM
PAM, PAM Analytics’ innovative asset management decision support tool, uses predictive analytics to meet this challenge by helping organisations adopt more proactive asset management policies. It models the dynamic risk (probability) of asset failure to mitigate the risk of future asset failure and extend asset lifetimes. Two applications of PAM are identifying pumps at greatest risk of imminent failure and identifying the locations of pipes at greatest risk of suffering water leakage and the times of greatest risk.
PAM bridges the gap between asset managers and maintenance managers. The operational module provides immediate cost savings, and the tactical and strategic modules provide insight and understanding into asset failure, so enabling cost savings at these levels to be made.
The upper button on the left (PAM Introduction) provides introductory information on PAM and the lower button (PAM Modules) has detailed information on each PAM module.
PAM is innovative in a number of ways, including:
Complete predictive asset management system. An integral part of PAM is gaining a thorough understanding of the data and preparing the data for the analysis and modelling - essential requirements for successful analytics projects and comprehensive modelling systems.
Uses predictive analytics. Most if not all asset management systems use business intelligence rather than predictive analytics. There is a fundamental difference between business intelligence and predictive analytics. Business intelligence looks backwards to report what has happened but does not provide any learning from this history as to what may happen in the future whereas predictive analytics uses historical data to develop predictive models for reporting what is likely to happen under a range of different scenarios. PAM uses predictive analytics and can therefore be used to gain insight and understanding into the causes of asset failure.
Models the risk of asset failure as a dynamic phenomenon. PAM models the risk of asset failure as a dynamic probability. Since current asset management systems only use static predictors, the calculated the risks of failure do not show how the risks change as assets are used and maintained. This may explain why most asset management policies are exclusively reactive or almost exclusively reactive, with all the consequent effects of increased asset management costs, low asset availability and poor customer service.
Models each asset as a unique entity. PAM models each asset as a unique entity with its own risk of failure profile. Models that only have static predictors generate the same risk of failure profile for all assets with the same value for each predictor.
|
|